Colour forecasting of the future
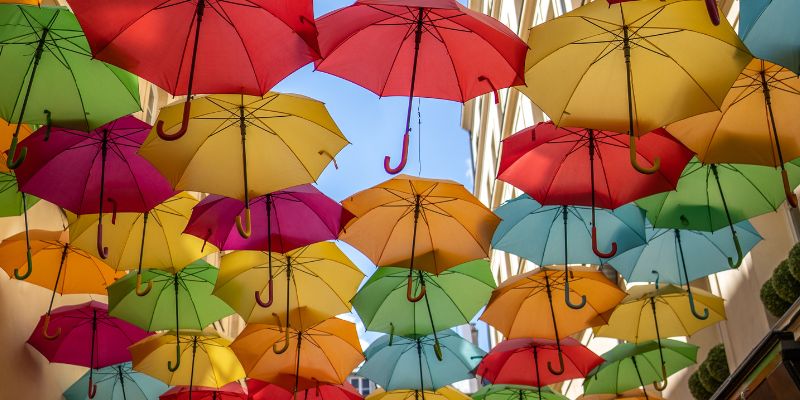
Colour forecasting is an important tool in the textile and retail industry; it informs the trends and decisions of future design seasons which can be essential to retailers.
There is concern, however, about whether colour forecasting can accurately predict consumer preferences so retailers sell what the customer actually wants or whether it operates as a sales tool to encourage consumers to buy more frequently as the trending colour will always change, encouraging the consumption of products that they may not want or need.
Professor Stephen Westland (Professor of Colour Science and Technology) and Peihua Lai (PhD Student, School of Design) analyse this phenomenon in the upcoming book, Bloomsbury Encyclopedia of World Textiles, through their contributing chapter titled ‘Data-Driven Colour Forecasting.’ In this article we extract the highlights from their chapter.
A brief history of colour forecasting
Colour and trend forecasting was a natural response to the requirements of design-manufacturing supply chains and the pressure to produce goods that consumers would want to buy in a timely manner. Modern colour forecasting agencies to service the requirements of manufacturers and retailers flourished and employed systematic analysis, involving several qualitative methods which nevertheless depend upon colour experts and forecasters’ intuitions. At the beginning of the 21st Century the concept of Colour of the Year was introduced by Pantone (Gijsenij et al., 2021) and today many companies produce their own colour of the year.
New supply-chain models
Traditionally the supply chain from design conception to retail purchase was around 18 months. This necessitated a trend forecasting business that was able to provide guidance about colours and styles. Some have argued that colour forecasting is essential to the textile and apparel industry and benefits manufacturers, retailers and consumers by enabling the right garments to be delivered in the rights styles and colours at the right price. However, an alternative view is that colour forecasting encourages waste and over-consumption.
Large numbers of unsold dead stock finds its way to landfill sites due to the difficulties in recycling many garments. The changes in technology in the 21st Century support new manufacturing models which allow agile manufacturing systems and this is resulting in a substantial reduction in the traditional 18-month cycle. In some cases the time between manufacture and retail is as little as three weeks. In one potential model for future manufacturing garments are sold before they are even manufactured. In such a scenario there is no deadstock because nothing is actually manufactured before it has already been sold (though the end-of-life outcomes may still remain an issue).
Automatic production methods in the fashion supply chain are shortening the forecasting duration and product lifecycles (Turker and Altuntas, 2014). As retail-supply chains shorten and we move towards a new manufacturing model with vastly reduced timescales this raises the question of whether traditional colour forecasting processes can still operate what the future of colour forecasting needs to be. Indeed, it may even raise the question of whether colour forecasting is needed at all.
Data-driven colour forecasting
Increasingly, methods are being developed that automate colour forecasting processes. These methods often use algorithms that use machine learning which is a technique that is frequently used in the pursuit of artificial intelligence. For example, clustering methods can be used to automatically work out what the dominant colours in an image are. One limitation of this simple algorithm is that often the background colour is the most frequent colour in an image but the background may be of much less interest to the user than other objects such as garments. The result of applying a simple clustering method and a more sophisticated approach, where a people-detection algorithm has been used to crop the image to the area that is likely to be of interest before the application of clustering (Lai and Westland, 2020). The colours that are from the more complex approach are more representative of the garments than those obtained from simple clustering.
It is likely that a human would be able to effortlessly extract the garment colours more accurately than the machine algorithms that have just been referred to. However, the advantage of the algorithms is that they can process thousands (indeed, millions) of images in a few hours and that is far beyond the capability of any person. Analysis of thousands of images from fashion shows can generate real-time colour reports.
Future directions
One argument against colour nowcasting is that it might allow colour trends to be identified and to change on a daily basis. Could this be a driver for fast fashion and lead to wasteful consumption? Certainly, this argument had already been used against the current colour forecasting industry which produces colour trends that change several times a year. One counter-argument is that just because we can measure colour trends on a daily basis doesn’t mean that they would necessarily change that often. Indeed, the use of data-driven colour forecasting might lead us towards a world where colour trends change less frequently when they are directly informed by consumer behaviour rather than when they are based on expert-driven intuition that is encouraged to artificially refresh colour trends several times a year. It is likely, however, that there will always be a role for experts even in an AI-powered future. The emerging challenge is how to ensure that these experts work with data-driven methods to allow the benefits of both human and machine insights.
Extracted from: Bloomsbury Encyclopedia of World Textiles: Data-Driven Color Forecasting (Stephen Westland and Peihua Lai Leeds Institute of Textiles and Colour, University of Leeds)
References
- Gijsenij, A., Vazirian, M., Kirchner, E., Spiers, P., Lai, P., Westland, S. and Koeckhoven, P., 2021.
- Analyzing a decade of Colors of the Year. Color Research & Application, 46 (1), 258-270.
- Turker, D. and Altuntas, C., 2014. Sustainable supply chain management in the fast fashion industry.
- An analysis of corporate reports. European Management Journal, 32 (5), 837-849.
- Lai, P. and Westland, S., 2020. Machine learning for colour palette extraction from fashion runway.
- images. International Journal of Fashion Design, Technology and Education, 13 (3), 334-340.